· Geography · 4 min read
Spatial Interpolation: Enigma Behind Mapping Patterns
Spatial interpolation helps create maps by estimating unknown values from known ones. Discover the fascinating process behind turning scattered data into cohesive patterns.
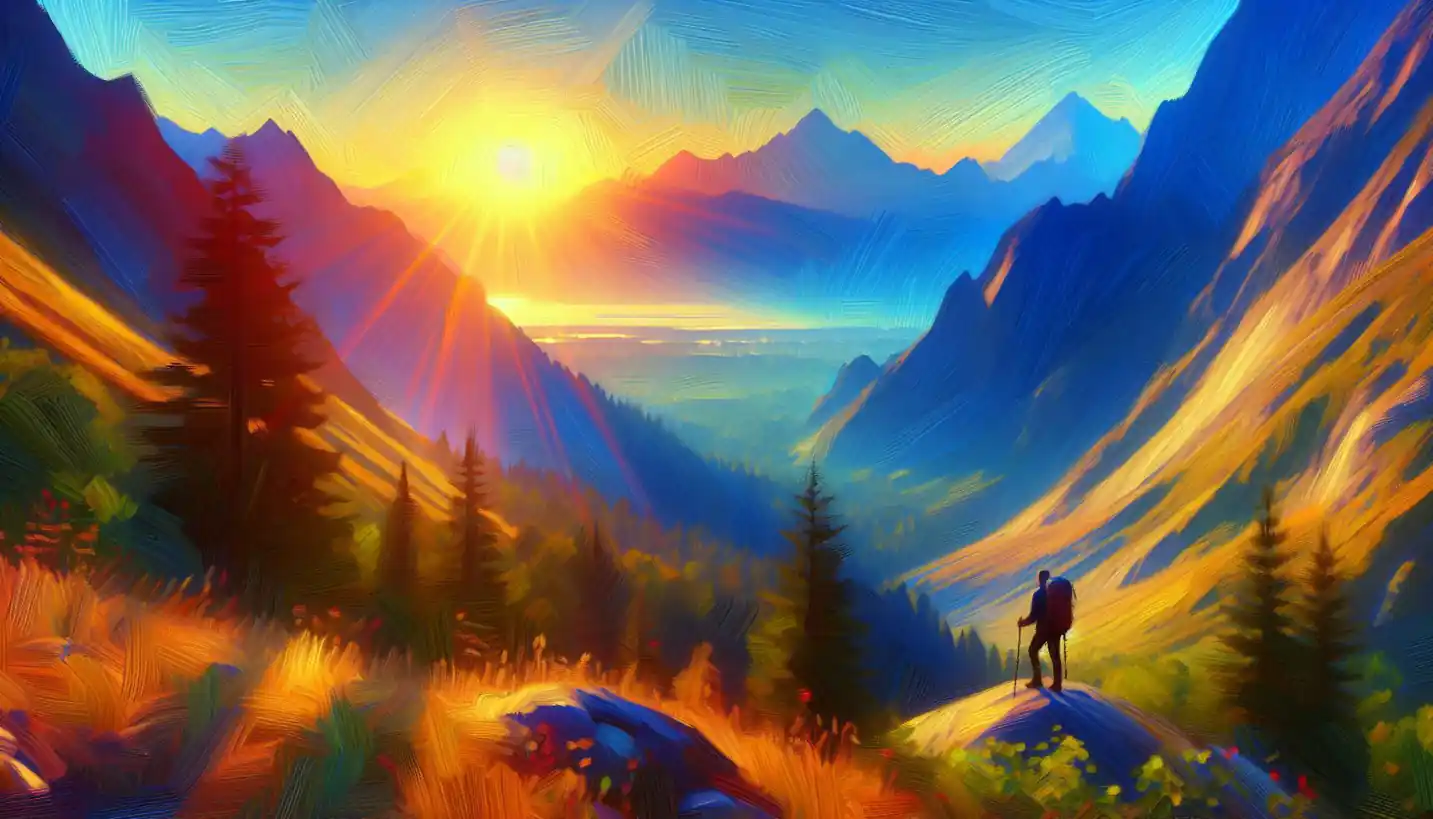
Spatial interpolation might sound a bit technical, but it’s all about creating a picture from dots that are often scattered far and wide. Think of it like connecting the dots in a puzzle to create a map of what’s between them. This technique is at the core of spatial analysis in geography, helping us understand everything from predicting the weather to planning cities.
Understanding how we fill in the gaps is key to many applications, so let’s dive into the world of spatial interpolation and why it’s so fascinating.
Bridging the Gaps: What is Spatial Interpolation?
When you look at a weather map showing temperatures across a region, you’re actually viewing a masterpiece of spatial interpolation. This process uses known data points—such as various weather stations reporting their readings—to estimate values in locations where data isn’t directly available.
Imagine you’re looking at a spread-out garden. You only have information about specific plants here and there. Spatial interpolation helps you imagine how the entire garden looks based on the few plants you’ve seen.
Why Interpolation Matters
Spatial interpolation is crucial because, in many cases, obtaining data for every single point on earth is simply unfeasible. Whether we’re examining soil quality, air pollution, or even real estate prices, gathering data can be time-consuming and expensive. Interpolation lets us make educated guesses about these unsampled areas, so planners and scientists can make informed decisions.
The Art and Science of Interpolation
Now, let’s get into some methods used to perform spatial interpolation. There are different ways to connect those dots, each with its own strengths and quirks. Here are a few popular ones:
Kriging
Kriging is like the Swiss army knife of spatial interpolation. It doesn’t just consider the distance between points but also their spatial arrangement. This method is fancy, but very powerful, providing estimates that come with a measure of confidence. It’s often used in fields like mining and meteorology.
Inverse Distance Weighting (IDW)
The simple idea behind IDW is “the closer, the more influence.” Points that are near carry more weight in determining unknown values. It’s straightforward and great when you have a fairly uniform distribution of data points.
Spline Interpolation
Think of spline interpolation like stretching a smooth elastic band through your known data points. It creates a smooth surface and is often used where a gentle gradation of values is expected, like in elevation models.
Real-World Examples and Applications
Spatial interpolation isn’t just an academic exercise. It’s a tool with real-world implications.
Weather Forecasting
Meteorologists use spatial interpolation to predict weather patterns. By taking readings from various weather stations, they can interpolate data to cover entire regions, forecasting storms and sunshine alike.
Environmental Monitoring
From tracking pollution levels to monitoring deforestation, spatial interpolation helps fill in the dots on how human and natural actions are shaping the planet.
Urban Planning
Cities are growing fast, and planners need to make quick, informed decisions. Spatial interpolation can help estimate demographic trends, guiding where to build schools, parks, or public transit.
Challenges and Considerations
Spatial interpolation isn’t magic. It has its challenges and limitations. The accuracy of interpolation depends significantly on the method used and the quality of known data points. If data is sparse or clustered unevenly, the interpolation might be less reliable. It’s a reminder that sometimes more data is required to draw a clearer picture.
Sparking Curiosity: The Future of Interpolation
What’s next for spatial interpolation? As technology advances, so does the ability to handle complex datasets and improve interpolation techniques. With the rise of machine learning, there’s potential for even smarter ways to fill in those gaps.
But questions remain. How do we accurately capture the diversity of environments across the planet? Can we make interpolation more accessible and understandable to those outside specialist fields?
These questions spark curiosity, inviting a new generation of geographers and data scientists to explore innovations in spatial interpolation. The future promises more accurate maps, more detailed analyses, and better-informed decisions.
Conclusion: The Magic of Filling in the Blanks
Spatial interpolation might seem technical, but remember, it’s all about creating stories and images from scattered pieces of information. It brings clarity to chaos, gives form to the formless, and guides us to understand the world in a way that pure raw data cannot.
By mastering ways to fill in the blanks, we not only improve how we see our world today but open doors to how we’ll interact with it tomorrow. Whether from weather maps or urban planning, the art of connecting those dots reveals the hidden patterns that shape our lives.
Through spatial interpolation, that hidden geography becomes accessible, tangible, and utterly fascinating.