· Geography · 4 min read
Spatial Autocorrelation: Understanding Patterns in Geography
Spatial autocorrelation examines how geographic elements are related spatially. Discover how this concept reveals patterns and clustering in data.
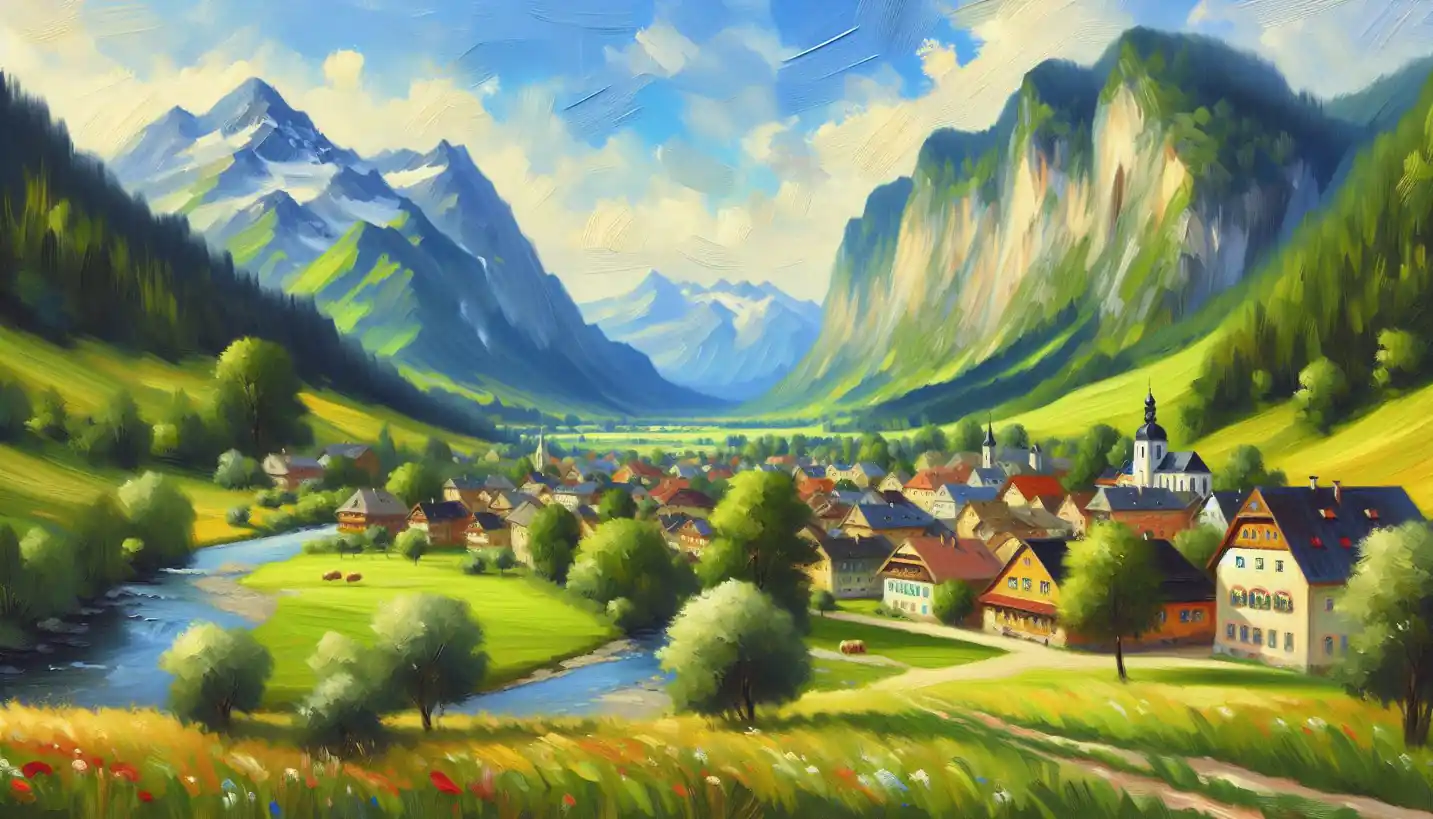
Have you ever noticed how certain things in a neighborhood seem to cluster together? Like how upscale cafes often group in trendy districts or how certain wildlife species tend to inhabit the same regions? This isn’t just a coincidence but a fascinating phenomenon in geography known as spatial autocorrelation.
What Is Spatial Autocorrelation?
To break it down, imagine spilling a bag of marbles on the floor. If the marbles roll into clusters instead of scattering evenly, those clusters are similar to what geographers call spatial autocorrelation. It’s basically a measure of how much nearby locations resemble each other. In simple terms, spatial autocorrelation helps us understand if things are ‘clumpy’ or ‘spread out’ on a map.
Positive and Negative Spatial Autocorrelation
When geographers talk about positive spatial autocorrelation, they’re referring to a scenario where similar values cluster together in space. For instance, think of a neighborhood where all the houses have similar architectural styles. On the flip side, negative spatial autocorrelation occurs when dissimilar values are found near each other, like alternating patches of forest and grassland.
Why Does Spatial Autocorrelation Matter?
Spatial autocorrelation isn’t just an academic curiosity. It plays a crucial role in various fields. Urban planners, for instance, use it to determine how land values increase around certain amenities like parks or schools. Environmentalists might apply it to study the distribution of species and their preferred habitats.
Real-world Applications
Consider public health. During an outbreak, knowing which areas show high positive spatial autocorrelation of disease cases can help authorities target interventions more effectively. Similarly, economists might analyze spatial autocorrelation in income levels to address regional inequalities.
Tools to Measure Spatial Autocorrelation
Geographers have developed several techniques to measure spatial autocorrelation. One popular method is Moran’s I, which helps to quantify the degree of spatial autocorrelation in a set of spatial data. If Moran’s I value is close to +1, it indicates a strong positive spatial autocorrelation. A value near -1 signals strong negative spatial autocorrelation, while a value around 0 suggests no spatial autocorrelation at all.
Other Methods
Apart from Moran’s I, there’s Geary’s C, another statistical tool that serves a similar purpose. Geary’s C emphasizes differences between spatial pairs, providing a complementary view to Moran’s I.
Delving Deeper: The Role of Distance
At the heart of spatial autocorrelation lies the concept of distance. The fundamental idea is that things located closer together often have more in common than things that are far apart. In geography, this principle is a reflection of Tobler’s First Law, which states, “Everything is related to everything else, but near things are more related than distant things.”
Distance Decay
This idea ties into the concept of distance decay, where interactions between locations decrease as the space between them increases. Think about how frequently you visit a local park versus one that’s several miles away. Similarly, spatial autocorrelation often weakens as distance grows.
Challenges and Considerations
However, measuring spatial autocorrelation isn’t without its challenges. Geographical phenomena are complex, and patterns can change based on the scale of analysis. For instance, a study of vegetation might show different patterns when viewed from a satellite compared to a ground-level inspection.
Scale and Modifiable Areal Unit Problem (MAUP)
The scale of observation significantly influences our perception of spatial patterns. This ties into what geographers call the Modifiable Areal Unit Problem (MAUP), where statistical results can vary based on how data is aggregated. Imagine analyzing climate data by country versus by continent; the patterns may look different.
Exploring Future Directions
Spatial autocorrelation is a gateway to deeper geographical analysis. It raises intriguing questions about the forces shaping our landscapes. How does urban development influence natural habitats? What are the impacts of climate change on regional farming patterns? As technology advances, our ability to analyze these questions will improve, providing more precise and insightful answers.
The Impact of AI and Machine Learning
With the rise of AI and machine learning, new tools are emerging to handle complex spatial data, offering fresh ways to study spatial autocorrelation. These technologies promise to unravel even more intricate patterns within datasets, enhancing our understanding of the world around us.
Conclusion
Spatial autocorrelation might sound like a mouthful, but it’s a simple yet powerful concept that helps explain the spatial structure of our world. From understanding urban landscapes to tackling environmental challenges, its applications are as varied as they are vital. As we continue to harness technology, our grasp on spatial autocorrelation will deepen, unlocking new possibilities for exploration and discovery.
Whether you’re curious about geography, urban planning, or environmental science, spatial autocorrelation offers a window into the patterns that define our surroundings. Next time you notice those clusters of similar traits, whether in cities or nature, you’ll know there’s a fascinating story of spatial autocorrelation behind them.