· Computer Science · 4 min read
Data Augmentation: Unlocking the Potential of Artificial Intelligence
Data augmentation enhances AI capabilities by expanding datasets, offering more robust learning opportunities.
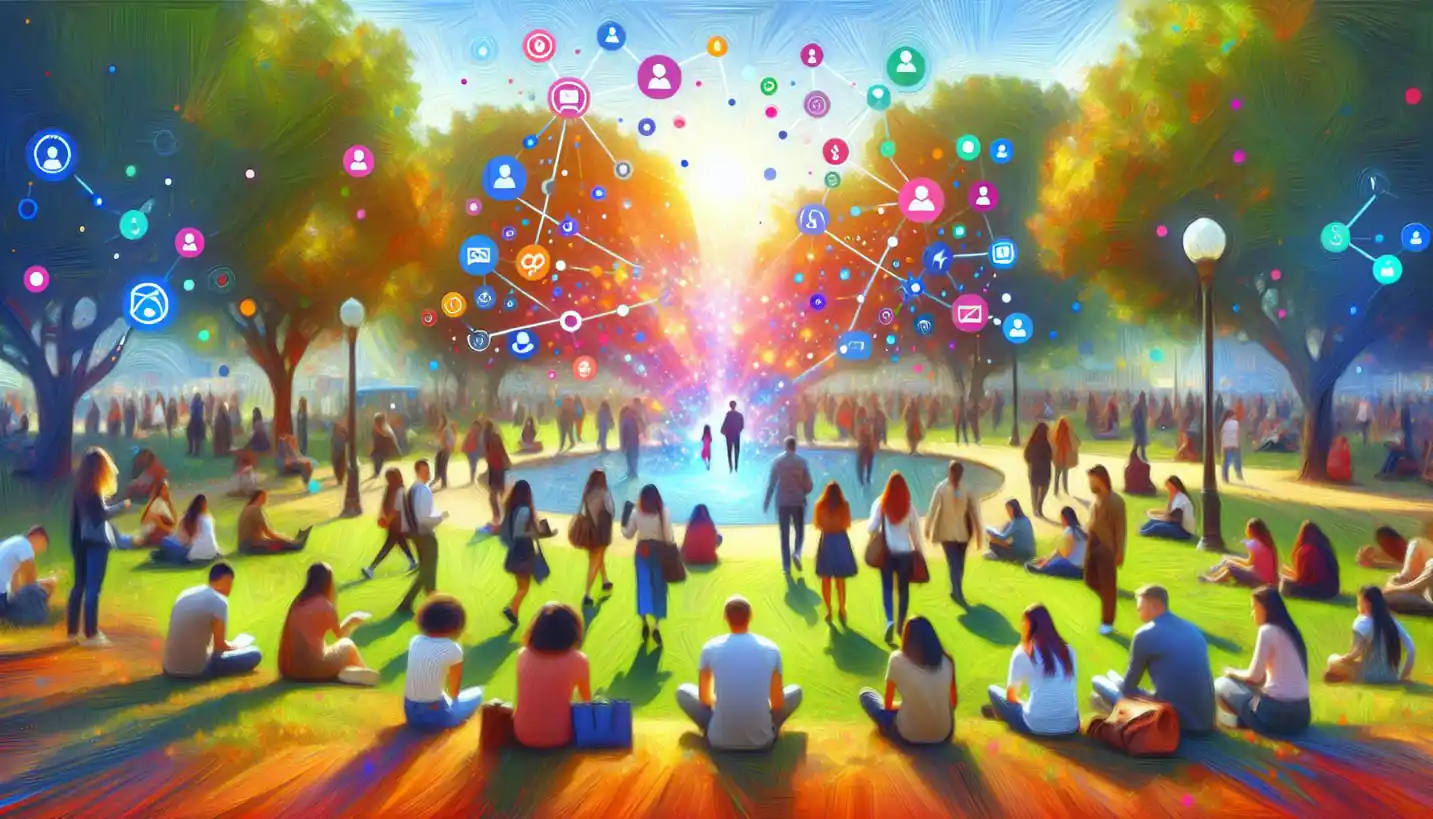
Stepping into the world of Artificial Intelligence feels a bit like entering a futuristic wonderland. Everywhere you look, machines are learning to think like us, but one major challenge remains: they need vast amounts of data to truly understand the world. Imagine trying to learn a new language with only ten phrases instead of thousands. That’s where data augmentation comes in, acting like a magic wand to transform and multiply data, giving AI the ability to learn more accurately.
What is Data Augmentation?
Data augmentation involves flipping, rotating, cropping, and adjusting what already exists to create new, yet similar, data. Think of it like taking a single family photo and using different filters and angles to make dozens of variations. This process is essential in training AI models, especially when gathering new data is difficult or expensive.
Why is it Important for AI?
Data is the lifeblood of AI, but high-quality data is not always readily available. When you need machines to recognize faces or distinguish between cats and dogs, having a broader set of examples makes the AI smarter. By augmenting data, we can inject diversity into our datasets, allowing models to generalize better and make more accurate predictions.
How Does Data Augmentation Work?
In simplest terms, data augmentation modifies existing data. For images, this could mean altering the brightness or contrast, cropping, or even converting to grayscale. For text, it might involve replacing words with synonyms or reordering sentence structure. Each transformation provides a new perspective, helping the AI understand different aspects of the data.
Example in Image Processing
Imagine you’re training an AI to recognize apples in photos. With limited pictures, you might only capture red apples from one angle. Data augmentation lets you stretch those few pictures into a treasure trove of images—green apples, upside-down apples, apples with different lighting conditions—broadening the AI’s understanding.
Beyond Images: Text and Audio
Data augmentation isn’t just for images. In natural language processing, for instance, you might swap words with synonyms or introduce small grammatical errors to enrich the dataset. Similarly, in audio processing, changing pitch or speed can create diverse sound samples, enabling AI to recognize different accents or pronunciations.
The Role of Technology
Advancements in technology help streamline the data augmentation process. Cutting-edge tools automatically generate augmented data, reducing the time and effort required from researchers. AI models can now be trained faster and with fewer resources, accelerating their deployment in real-world applications.
The Human Element
Despite technological advancements, the human touch is crucial. Creatively thinking about how to effectively augment data relies on understanding not just the technology, but also the nuances of what you want the AI to learn. Crafting the right data augmentation strategy can be pivotal for success.
Challenges and Considerations
While data augmentation is powerful, it’s not without pitfalls. Over-augmenting or introducing inappropriate transformations can confuse rather than help an AI model. Striking the right balance is key—imagine suddenly changing all apples to oranges. The AI might learn something, but it won’t be what you intended.
Future Directions in Data Augmentation
As AI continues to evolve, data augmentation will remain a vital tool in the researcher’s toolkit. With the rise of deep learning and neural networks, more sophisticated techniques are emerging, such as using AI to augment data automatically. These advances will open doors to new possibilities, ensuring AI models are more robust and versatile.
The Broader Impact
Emphasizing the importance of data augmentation reflects on the larger goal of AI: creating machines that understand and interact with the world like humans do. Enhanced data results in more reliable models, whether applied in healthcare, finance, or any other field. It’s a foundational step towards making AI safer and more efficient.
Conclusion
From expanding the horizons of what AI can recognize to accelerating its learning process, data augmentation is a silent powerhouse driving the field forward. By creatively enhancing existing data, we unlock more potential from AI, bringing us closer to realizing its full capabilities. As technology evolves, the ways we manipulate and augment data will continue to adapt, crafting smarter, more perceptive machines for the future.
In the end, data augmentation is about making the most of what we have—much like turning a single photograph into a gallery of experiences. It’s a journey of expanding possibilities, paving the way for AI to understand and navigate our complex world more completely.