· Economics · 4 min read
Understanding Variance Inflation Factor: A Key Concept in Econometrics
Variance inflation factor is critical, measuring multicollinearity in econometric models and ensuring your data remains robust and reliable. Understand the importance of the variance inflation factor, a sentinel guarding against the pitfalls of misleading econometric conclusions.
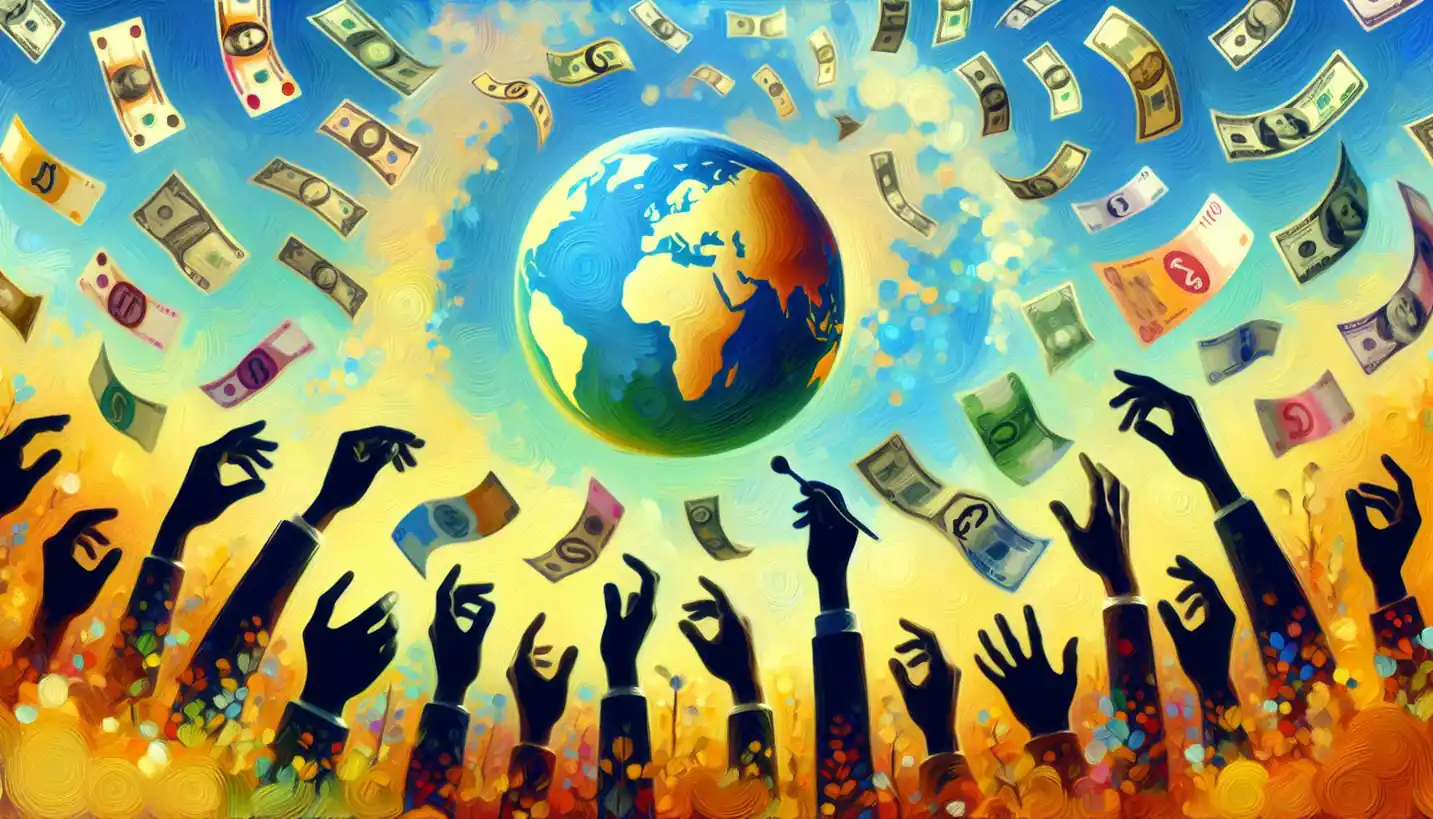
Have you ever tried juggling multiple tasks at once, only to find things getting tangled? In econometrics, a similar concept exists called the Variance Inflation Factor, or VIF, which helps us untangle the complexities of multicollinearity in regression models. Let’s dive into what VIF is and why it’s so vital.
What is Variance Inflation Factor?
Imagine that you are baking a cake, and you decide to add more and more of each ingredient without a recipe. Soon, it becomes nearly impossible to tell which ingredient affects the taste most. VIF is like a chef’s guide that helps in identifying what might be overshadowing other flavors, or in statistical terms, which variables are interconnected in ways that can skew results.
In econometrics, we frequently use regression analysis to understand relationships between variables. However, when these independent variables are highly correlated, they can lead to misleading insights. This is where VIF comes into play. It measures how much the variance of an estimated regression coefficient is increased because of collinearity.
How Does VIF Work?
Think of VIF like a magnifying glass for relationships between variables. It examines each predictor variable within the context of all the others. If any variable increases the VIF value, it signals a potential multicollinearity issue.
The VIF of a variable is calculated using a simple formula:
[ VIF = \frac{1}{1 - R^2} ]
Here, ( R^2 ) is the coefficient of determination of a regression of one predictor on all the others. A VIF value of 1 suggests no correlation among variables, while a value exceeding 5 or 10 indicates a somewhat problematic collinearity.
Why is VIF Important in Econometrics?
In the world of economics and statistics, precision is key. Multicollinearity might not affect the model’s ability to make predictions, but it can seriously hamper the interpretation of your model. Consider a medical researcher trying to understand the impact of various lifestyle factors on heart disease. If variables such as exercise and diet are closely correlated, separating their individual effects becomes challenging. Reducing this confusion is exactly what VIF helps with.
Econometric models are often complex, attempting to consider multiple variables at the same time. VIF becomes an essential diagnostic tool, ensuring that the insights drawn are both reliable and valid.
How to Tackle High VIF?
Finding a high VIF in your analysis is like discovering your car’s engine is overheating. You need to address it before moving forward. Here are a few strategies:
Removing Variables
One straightforward solution is to remove one or more of the closely correlated variables. If two ingredients in your recipe are overwhelming the dish, removing one can balance the taste.
Combine Variables
Sometimes, combining variables into a single factor can solve the multicollinearity problem. It’s like blending spinach and kale into a single “greens” category in a smoothie.
Standardize Data
Transforming data by standardizing or normalizing it can sometimes reduce VIF. Adjusting the scale can bring clarity, making the relationships between variables less murky.
A Real-World Example
Let’s envision a real-world scenario involving housing market analysis. Economists might study how factors like interest rates, average income, location, and unemployment affect housing prices. It’s quite plausible that income and location are correlated with the interest rates, leading to high VIF values for those predictors.
By using VIF, analysts can recognize this issue and make necessary adjustments, perhaps by creating a composite variable or focusing on the most impactful factor, leading to a clearer understanding of which variables truly drive the housing market.
Future Research and Implications
The topic of multicollinearity and VIF in econometric models remains a vibrant area of research. As datasets grow more complex with ever-increasing variables to consider, understanding the intricacies of these relationships is crucial. Researchers continue to explore novel methods to detect and manage multicollinearity more efficiently.
With the expansion of machine learning and artificial intelligence in econometrics, the future holds exciting opportunities and challenges in managing multicollinearity. VIF will likely remain a staple tool but might evolve through integration with advanced techniques.
Conclusion
You’ve now glimpsed into the world of econometrics through the lens of the Variance Inflation Factor. It’s more than a simple mathematical concept; it’s a critical tool that helps economists and statisticians untangle the complexities of multicollinearity, leading to more accurate and meaningful models.
Whether you’re diving into econometrics for the first time or seeking to deepen your understanding, appreciating the role of VIF can guide you to clearer, more precise insights.
Questions about how VIF might impact your next project? The world of economics is full of fascinating stories and discoveries waiting to unfold. Keep exploring and questioning, for curiosity is the catalyst of knowledge.