· Economics · 4 min read
Autocorrelation: Cracking the Mystery in Econometrics
Autocorrelation occurs when data points in a series are interdependent. Crack this econometric mystery to ensure accuracy in model predictions and analysis.
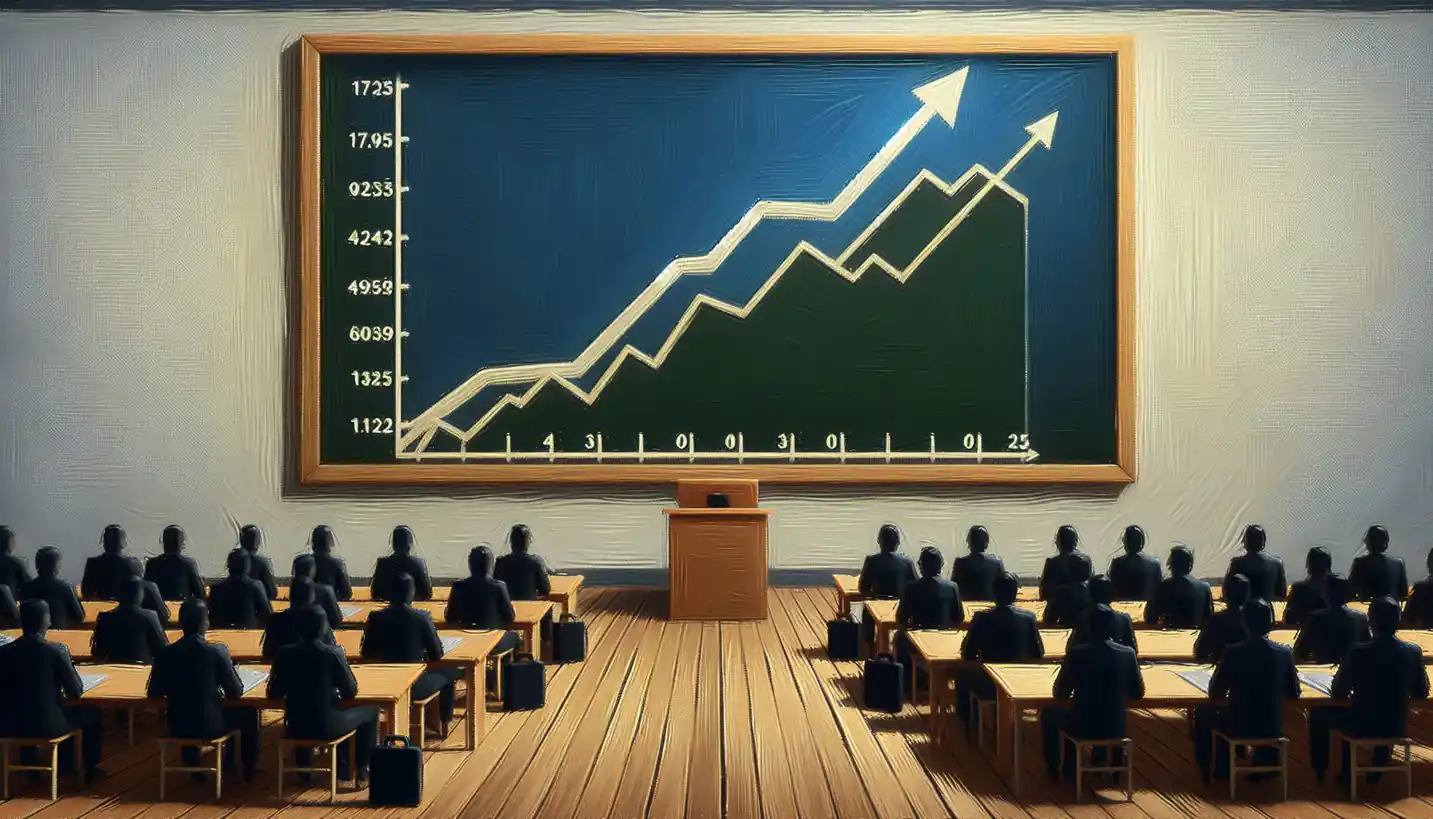
Once upon a time, economists stumbled upon a curious phenomenon that seemed to whisper secrets about repeating patterns in data. This mystical occurrence is known as autocorrelation. Like finding echoes in a canyon, autocorrelation reveals how data points in a series are related to one another over time. Let’s dive into this concept, unravel its mysteries, and see why it plays such an essential role in econometrics and economics.
What is Autocorrelation?
Imagine you’re listening to your favorite song, and you notice that certain beats repeat themselves at regular intervals. Autocorrelation is a bit like that in the world of data analysis. It occurs when successive data points in a time series are correlated with each other. In simpler terms, it means that what happens at one point in time is related to what happens at another.
In financial markets, for instance, the price of a stock today might have some connection with its price yesterday or last week. This relationship can help economists predict trends and make decisions about investments.
The Importance of Autocorrelation in Econometrics
Why does autocorrelation matter so much? Well, it’s because when we analyze economic data, we often assume that each data point is independent. However, if autocorrelation is present, this assumption falls apart. Ignoring these connections can lead to misleading results.
For example, imagine trying to forecast economic growth without considering how past growth affects future trends. Autocorrelation provides economists the tools to refine models, ensuring more accurate forecasts.
Detecting Autocorrelation: A Guided Journey
Spotting autocorrelation isn’t as simple as listening for echoes. Economists use statistical tests to detect it. A popular method is the Durbin-Watson test, which investigates the relationship between error terms in a regression model.
To put it simply, if the test reveals a pattern in the noise, autocorrelation might be present. Another method is the Ljung-Box test, which checks for randomness in a dataset, helping to confirm whether autocorrelation effects persist over time.
Real-Life Examples and Implications
Autocorrelation is not just an abstract concept; it shows up in real-life situations. For instance, consider the weather. If today is sunny, there’s a reasonable chance that tomorrow might be sunny too. In economics, autocorrelation can appear in GDP growth rates, where growth one year might influence growth the following year.
This brings us to the implications. If a government wants to implement a policy to boost GDP, understanding these patterns can provide invaluable foresight. Ignoring autocorrelation might lead to wrong policy decisions, potentially causing economic harm.
Fighting the Beast: Addressing Autocorrelation
Once detected, what’s the next step? Econometricians must adjust their models to account for autocorrelation. One way is through transformation techniques like differencing, where differences between consecutive data points are analyzed instead of the raw data.
Alternatively, adding lags to models can neutralize the effects, providing a clearer picture of cause and effect. Autoregressive models (AR) are often used, where past values are included as predictors to mitigate autocorrelation.
The Bigger Picture: Autocorrelation in Macroeconomics
Beyond individual stocks and weather forecasts, autocorrelation plays a vital role in macroeconomic analysis. Imagine central banks trying to control inflation. By understanding how past inflation rates affect future ones, policymakers can better tailor interest rates to manage economies efficiently.
Here, autocorrelation aids in modeling complex relationships between different economic variables, leading to well-informed monetary policies.
Sparking Curiosity: What’s Next in Autocorrelation Research?
The journey of understanding autocorrelation is far from over. Economists continue to explore its depths, searching for new patterns and connections. Some recent research delves into non-linear models that can capture more complex forms of autocorrelation. This could revolutionize the way we interpret economic cycles and financial crises.
Furthermore, with the rise of big data, researchers are developing sophisticated algorithms to detect and model autocorrelation in vast datasets, potentially unlocking hidden insights in economic trends.
Why Should You Care About Autocorrelation?
You might wonder, why should the average person care about autocorrelation? Well, anyone interested in economics, finance, or data analysis needs to grasp this concept. Recognizing the patterns and connections can help you make informed decisions, whether you’re investing in the stock market or analyzing economic policies.
Moreover, understanding autocorrelation fosters a deeper appreciation for the precision and complexity involved in economic forecasting. It’s a reminder that patterns in the seemingly random flow of data can tell powerful stories about the past and future.
Autocorrelation, with its echoes of the past affecting the future, is a fascinating concept that bridges our understanding of time, data, and economics. By recognizing and harnessing this phenomenon, we can pave the way for more accurate predictions and better decisions in a rapidly changing world.